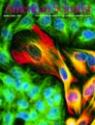
This Article From Issue
March-April 1998
Volume 86, Number 2
DOI: 10.1511/1998.21.0
Deconvolution of Images and Spectra. Second Edition. Peter A. Jansson, ed. 514 pp. Academic Press, 1997, $99.
This edited collection of chapters on various deconvolution topics is a revised and expanded version of Jansson's Deconvolution with Applications in Spectroscopy (Academic Press, 1984). Each chapter begins with a list of symbols and ends with an extensive list of references—both extremely helpful to the reader.
The first three chapters are by Jansson, who begins with an excellent background introduction giving definitions and properties helpful in using and understanding the remaining chapters. Chapter two discusses deconvolution of optical spectra and chapter three surveys traditional linear methods, including Van Cittert's iterative method, a matrix formulation and inverse filters.
Chapter four, "Modern Constrained Nonlinear Methods," also by Jansson, contains the first additions to the 1984 volume. There are expanded sections on ratio methods, the methods of Schell, Jansson, Biraud, Frieden (maximum likelihood) and Howard, alternating projection and Monte Carlo methods, and the use of neural networks. Jansson's method receives the most detailed discussion, and a new section on reviews and reference works appears.
"The Convergence of Relaxation Algorithms," by Paul Crilly, is entirely new. This chapter includes sections on error analysis, the mathematics of convergence, and experimental testing. In chapter six, William Blass and George Halsey discuss equipment requirements with the specific example of optical spectroscopy, including a discussion of the difficult problems of noise. Crilly joins Blass and Halsey in "Deconvolution Examples," which compares the iterative methods of Van Cittert, Jansson and Gold, and presents examples of high-resolution grating spectra, tunable-diode-laser spectra, Fourier transform infrared spectra, laser Raman spectra, high-resolution gamma-ray spectra and gas chromatographic peaks.
Another new chapter, "Deconvolution in Optical Microscopy," by Jason Swedlow, John Sedat and David Agard, covers image blurring in optical microscopy, data for image deconvolution, constrained iterative deconvolution and its uses, and other methods. "Deconvolution of Hubble Space Telescope Images and Spectra" by Robert Hanisch, Richard White and Ronald Gilliland is, of course, also a new addition. Hubble data are considered, with discussions of the telescope point-spread function, the Richardson-Lucy algorithm and adaptations, phase retrieval and blind deconvolution, spectral deconvolution, image restoration and photometry, and post-servicing mission performance. The last chapter, "Alternating Projections onto Convex Sets" by Robert Marks, is also new. It is a mainly mathematical discussion covering geometrical projections onto convex sets, convex sets of signals, and examples.
Two chapters by Samuel Howard, "Fourier Spectrum Continuation" and "Minimum Negativity-Constrained Fourier Spectrum Continuation," have been retained and renumbered. Robert Davies has been added as co-author (with Jansson) of "Application to Electron Spectroscopy for Chemical Analysis." Roy Frieden's "Maximum Probable Estimates of Spectra" (renamed and 7 pages longer) includes discussions of the physical model and degrees of freedom, the most probable object, a "white" object (maximum entropy), zero prior knowledge (maximum ignorance), estimators and noise.
This is an excellent practical handbook for using deconvolution with real data for which the positivity constraint is useful, such as in optical images and spectra. Noise is discussed throughout the book, along with various recommendations for solving specific problems. Figures are clear and the text is very readable. The chapters relate reasonably well, especially considering the number of authors, and it is helpful to have many examples of deconvolution for a variety of research problems. We are happy to have this book and recommend it to anyone involved in data analysis or signal processing which involves deconvolution.—Juliette W. Ioup and George E. Ioup, Physics, University of New Orleans
American Scientist Comments and Discussion
To discuss our articles or comment on them, please share them and tag American Scientist on social media platforms. Here are links to our profiles on Twitter, Facebook, and LinkedIn.
If we re-share your post, we will moderate comments/discussion following our comments policy.