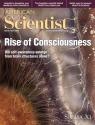
This Article From Issue
March-April 2025
Volume 113, Number 2
Page 66
Thinking about thinking is a common human passtime. Our sense of identity seems to drive our consciousness, but it can also make it feel like there’s a separate voice in our heads providing running commentary all day long, explains Alan J. McComas. In “Consciousness: The Road to Reductionism,” McComas describes how decades of neuroscience research have explored the emergence of how humans think and feel, and how conclusions about that process have morphed over time.
McComas argues for a reductionist approach, meaning that consciousness is a function of the brain, and can be explained by analyzing its parts. Interestingly, studies have repeatedly challenged our perception that we are in charge of our consciousness. Neural activity can precede our awareness of tasks that we seemingly decide to undertake, and memory can be activated before our realization of thoughts and ideas. McComas emphasizes that significant questions remain about how our brains create our subjective experiences, but new discoveries are helping us to understand ourselves.
The question of consciousness frequently comes up when people interact with one of the many chatbots now widely available. Devices ranging from smartphone digital assistants, such as Siri, to more recent bots such as ChatGPT, Gemini, and Claude, can seem like they are capable of intelligent responses, and perhaps some level of understanding. The artificial intelligence programming behind these devices is built on massive computing power and algorithmic sophistication that has been developed specifically to mimic human communications, so it’s no wonder that these devices can be convincing. But as Federico Fede and Viviana Masia describe in “The Manipulative Side of Chatbots and AI,” these programs are also acquiring techniques to use tone and rhetoric that can imitate deceptive and manipulative speech.
Fede and Masia study the restrictions and potential uses of large language models, or LLMs, the technology that allows chatbots to understand and produce natural language. LLMs recognize human patterns of language based on huge quantities of text. This analysis allows the devices to develop parameters that optimize their responses to different types of input. They can identify the broader contexts of sentences, and consider not just the words used, but also phrases, relationships, and boundaries between terms. As most people know, these abilities are far from perfect; chatbots still frequently give wrong answers and, what’s worse, will sometimes fabricate information.
Fede and Masia describe how awareness of the rules of rhetoric can aid users in remaining vigilant for when chatbots use manipulative language as they attempt to give more realistic responses. A chatbot may use implicit communication, presupposition, vagueness, or figurative language to color their responses. Implicit communication transmits information without it being stated and presupposition represents information that is taken for granted. Keeping an eye open for such usages can prevent users from putting too much stock in chatbot responses that employ them.
In prior issues of American Scientist, other authors have discussed how artificial intelligence has infiltrated research, either in data analysis, in generating figures, or even in preparing text. One author wondered when AIs would have to be listed as coauthors. Many journals have issued standards covering the use of AI in submitted papers. But these standards are evolving quickly, and scientists will need to keep pace.
American Scientist Comments and Discussion
To discuss our articles or comment on them, please share them and tag American Scientist on social media platforms. Here are links to our profiles on Twitter, Facebook, and LinkedIn.
If we re-share your post, we will moderate comments/discussion following our comments policy.