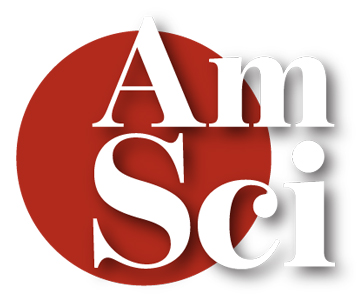
Thomas R. Sinclair
Thomas R. Sinclair is a visiting professor in agronomy@the University of Florida and an adjunct professor in crop science@North Carolina State University. Raised on a farm in Indiana, he received bachelor’s and master’s degrees from Purdue University and a Ph.D. from Cornell University. Sinclair researches ways interactions between plant physiology and the environment determine crop yield. Currently he is focused on plant traits that might increase drought tolerance. He has pursued studies in Argentina, Australia, Israel, Italy, Japan, the Netherlands, New Zealand, and, as a Ballard Fellow,@Harvard University. Sinclair has edited several books, including Principles of Ecology in Plant Production. He and his wife, Carol Janas Sinclair, recently finished the book manuscript Seeds of Change: History through Agriculture, Bread and Beer. Address: Crop Science Department, North Carolina State University, Raleigh, NC 27965. Internet: trsincl@ifas.ufl.edu