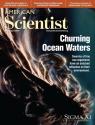
This Article From Issue
July-August 2024
Volume 112, Number 4
Page 246
HOW TO WIN FRIENDS AND INFLUENCE FUNGI: Collected Quirks of Science, Tech, Engineering, and Math from Nerd Nite. Chris Balakrishnan and Matt Wasowski. 320 pp. St. Martin’s Press, 2024. $30.00.
If you’ve never happened across a Nerd Nite, it is an in-person, public phenomenon that began in Boston in 2003 with the goal of bringing curious, “nerdy” people together to learn and be entertained by 20-minute presentations on a variety of science subjects, usually at a bar, often with plenty of imbibing. Now, Nerd Nite is global, with about 200 presentations a month taking place.
Chris Balakrishnan and Matt Wasowski, cofounders of Nerd Nite and the editors of the new book How to Win Friends and Influence Fungi: Collected Quirks of Science, Tech, Engineering and Math from Nerd Nite, state that one of their founding mottoes was, “It’s like the Discovery Channel with beer.” The book is a curated collection of short essays written by “70 of [their] favorite nerds from around the world.” Some of these contributing nerds are career scientists, some are medical doctors, some are professional science communicators or educators, and some have been or are in charge of Nerd Nite events. All of them have presented at a Nerd Nite over the past 20 years, and they are generally curious people with extensive knowledge on interesting niche topics related to science and technology that they are excited to share.
How to Win Friends and Influence Fungi is divided into topical sections that begin with brief, humorous, personal introductions from one of the editors, giving us a glimpse into their own special interests and leanings in science. Sections include “Creature Features,” about different weird creatures on Earth; “Mmm . . . Brains,” which explores topics in psychology and neuroscience; “Death and Taxes (But Really, Just Death),” a section about—you guessed it—death; and “Careers,” where presenters share the vast array of paths within science.
Each section is a grab bag of topically relevant expositions from different authors hailing from the various locales worldwide where Nerd Nites occur. While it is hard to translate the experience of an in-person event onto the written page, the care that Balakrishnan and Wasowski put into choosing contributors is obvious in the throughline of humor and clarity of the writing, as well as in the diversity of specialties and research interests on display.
Each section is a grab bag of topically relevant expositions from different authors hailing from the various locales worldwide where Nerd Nites occur.
The variety of content and perspectives ensures that just about any reader will find new ideas to ponder. Have you heard the phrase “phonotactic constraint”? Mari Sakai, a linguist and speech consultant who works to improve communication in global settings, explains it as a language’s “preference for putting the sounds together.” These changes in sound that occur in speech patterns are an intrinsic aspect of language that influences how we speak, which Sakai explores in her fascinating essay about accents.
Paula Croxson, a neuroscientist, science communicator, and storyteller who apparently gets terrible hangovers, may or may not help you cure the hangover you incur while reading this book and enjoying a cold adult beverage. Her essay details what happens when you ingest alcohol, how the alcohol is then broken down, and the subsequent histamine response that occurs in some people who don’t make enough aldehyde dehydrogenase to break down the alcohol, potentially causing a hangover.
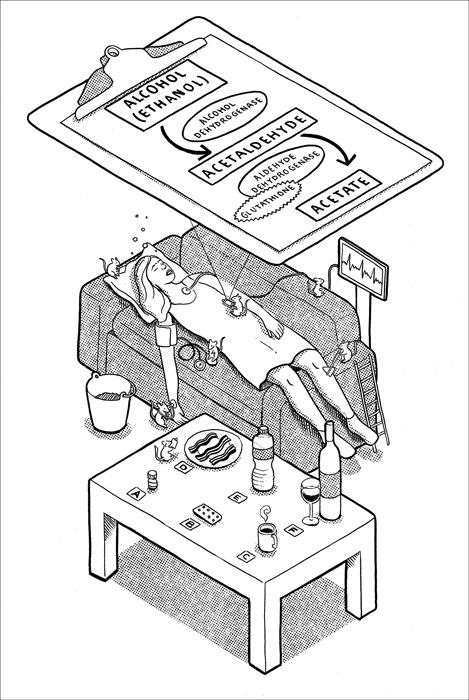
Illustration by Kristen Orr.
The terrible jokes introducing the section “Bodily Fluids” will make almost any reader laugh (my own sense of humor hasn’t aged since I was 12). And if you, too, are prone to laughing at punny jokes like, “Mucus pun? Don’t even goo there ... ,” do not take a sip of your beverage before turning to that particular page. Just put the drink down right now. Once safely immersed in the section’s material, you will have access to not only the world’s oldest joke, but also to more than you ever wanted to know about bladder control and poop in space. But this section isn’t just about potty humor. Brendan Byrne, in his essay about “dealing with poop and pee in space” writes, “NASA’s Trash to Gas technology would take that waste and incinerate it, creating valuable water that could also be broken down into hydrogen and oxygen, two key sources for rocket fuel.” This developing technology could then be used here on Earth as well, to great benefit.
Throughout the book are wonderful illustrations drawn by Kristen Orr, a brilliant artist who studied science and design, which enhance the reading experience. What, you can’t picture how the connections between your bladder, brain, and legs keep you from peeing? Don’t worry; there’s an image to help you understand how the system works and how it might get confused. In the section “Doing It,” Orr’s comical, yet potentially NSFW drawings (though it really depends on how you interpret cartoons of stuffed-animal monkeys without genitals) are a delightful addition to primatologist and science comedian Natalia Reagan’s essay on pansexual primates. Orr also wrote a chapter about nonmammalian milks, which is supplemented by a graphic that will make you want to double-check the label on the milk carton in your refrigerator to make sure that it comes from your preferred milk producer and not a pigeon or caecilian.
This wonderfully entertaining essay collection contains something (probably several somethings) for any science-curious reader. For those who are more hesitant, I daresay the essays will make learning about science enjoyable—and even fun!—thanks to the book’s style and approach to its subjects. How to Win Friends and Influence Fungi is the kind of book that you can sit down and read straight through in one sitting, or approach like an à la carte science menu, and the short essay format lends itself to shorter attention spans or to those moments when you have limited time but would prefer a quick read to an internet video. It is a book that can be read almost anywhere—especially while waiting at a bar for a friend, preferably while wearing a nerdy T-shirt.
American Scientist Comments and Discussion
To discuss our articles or comment on them, please share them and tag American Scientist on social media platforms. Here are links to our profiles on Twitter, Facebook, and LinkedIn.
If we re-share your post, we will moderate comments/discussion following our comments policy.